Use Machine Learning to Increase Streaming Efficiency
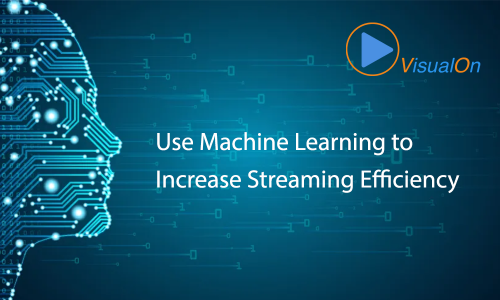
The adoption and proliferation of streaming video faces multiple challenges, most notably scale and quality. Artificial Intelligence (AI) and Machine Learning (ML) are increasingly being applied to perfect the streaming service and increase the delivery efficiency.
While video quality is increasing with the adoption of high-resolution, high-frame rate video such as HD, UHD & AR/VR, the volume of video data is also growing, and the cost of bandwidth. CDN transfers and storage associated with video consumption is increasing every year, which highly impacts the Quality of Experience and streaming costs. Nowadays, video compression software leverages AI and ML to automate video compression. An ML-based algorithm that efficiently, automatically and optimally configures the encoder to achieve best results based on the input contents.
Deploying and maintaining high-quality video services can be frustrating and cumbersome. It requires significant testing and troubleshooting to discover what is causing playback issues before you can even start to debug and qualify devices and streams. ML-powered automation tools can be used to pinpoint the exact moment when an issue occurs, and flag potential problems before they happen. It analyzes where rebuffering, frame drops or other errors occurred and marks those events for analysis and testing.
We are still at the early stage applying AI and ML to video streaming, especially live video streaming. Classifying, recognizing objects, and recognizing faces are tasks that AI and ML should manage in the future, which can help solve problems, from protecting privacy to improving the quality of user experience.